How over 20 publishers are jointly inventing digital publishing
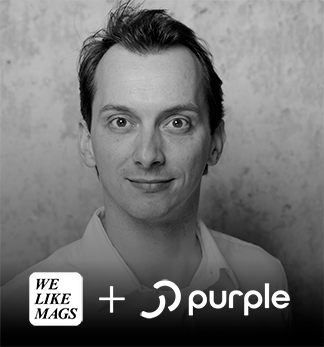
Audience Development Deep Dive Episode 5: Dr. Christoph Mayer
We Like Mags and Purple in conversation with Dr. Christoph Mayer, Partner and Managing Director AI & Data Science at the management consultancy Schickler
When media consultant Dr. Christoph Mayer is most frequently asked by various publishers, "How do we read the data so that we can align our products and newsrooms with it?" it makes sense to explore these questions together in a working group.
Improving the digital business model together - with DRIVE
Mayer's focus is on regional publishers that compete with each other in marginal areas, but are all confronted with the same problems and challenges - both technical and personnel-related. At the center of this is another question, namely: How do I turn users into convinced subscribers?
This is how the idea for the DRIVE project came about. The basic idea of DRIVE is simple: Only a joint approach can help against the overwhelming power of Google, Facebook & Co. Around 20 newspaper publishers such as NPG Ulm, DDV-Mediengruppe and Mittelbayerische Zeitung are involved in the project. Mayer and his team are collecting data for these customers. Every user action on the websites is stored and managed and analyzed at Schickler. Even if not every analysis is relevant for some of the participating publishers, they still benefit from the collected results of all of them.
Mayer calls this "large-scale experimenting and shared learning." All findings and results are available for all publishers to see. It's a very transparent process that also allows publishers to work at a speed they were not able to before, due to a lack of both human and technical resources. Added to this is Schickler's expertise, with a trained view from the outside, to read the data in such a way that it actually leads to the target.
Further links
What awaits you in this episode
00:36 How does Schickler advise media companies?
02:39 What publishers want - the BDZV Trend Survey 2022 by BDZV/Schickler
05:12 When do digital revenues start to make a noticeable difference?
07:41 Do personalized offers really prove to be a sales booster?
13:44 Content stream vs. e-paper
18:22 Look at the needs of your target group
26:51 How the DRIVE project is shared by 20 publishers
31:45 Do not measure in old KPIs
37:06 Media Time as a new Key Performance Indicator
42:53 What role do data science and AI play in DRIVE?
47:14 Why focusing on the top 5% of your users is important
49:59 Which subscriptions does Dr. Christoph Mayer have privately?
Christian Kallenberg [00:00:07]:
Welcome to the Audience Development Deep Dive from SPRYLAB Technologies and We Like Mags. Once again, sitting across from me today as co-host is Benjamin Kolb, CEO of SPRYLAB Technologies. Hello Benni.
Benjamin Kolb [00:00:21]:
Hello, Christian, and hello, Christoph.
Christian Kallenberg [00:00:24]:
Because Christoph is our guest today. Dr. Christoph Mayer, Partner and Managing Director AI and Data Science Practice at the consultancy Schickler. Hello, Christoph.
Dr. Christoph Mayer [00:00:35]:
Hello.
Christian Kallenberg [00:00:36]:
Christoph, I've just told you what you do for a living, but that sounds insanely great. What does that actually mean?
Dr. Christoph Mayer [00:00:43]:
Well, sometimes I'm still trying to figure that out myself, maybe. In the end, what we do at Schickler is: we bring deep understanding of processes, business models in the media industry, a lot in the publishing houses, along with data, data science, algorithms, data engineering and everything that comes with it. We always say "end-to-end view." The topics start with a business question, move on to analysis, algorithms, and so on, and end with a business question again. Mostly the question: What do I actually do with it now? And that is a bit of the overall picture that we support as a company.
Christian Kallenberg [00:01:20]:
And did I understand that correctly, Christoph? Your unit focuses primarily on media companies, or is that one part of many?
Dr. Christoph Mayer [00:01:29]:
Our focus as a whole company, Schickler in total, is on media companies. We work, I would say, on media companies at least 60%, 70% of it is for publishers. The rest is in radio, TV, book and a large part is really on publishers.
Christian Kallenberg [00:01:46]:
And how big is your area? So how many people work there?
Dr. Christoph Mayer [00:01:49]:
Yes, so Schickler all-in are about 30 people. The data area is currently six, next weeks seven, and in a few weeks almost ten.
Christian Kallenberg [00:02:01]:
This is an interesting growth.
Dr. Christoph Mayer [00:02:02]:
So I always say there are ten - a little bit ahead of time.
Christian Kallenberg [00:02:05]:
Yes, the future is always good. Can you talk about who your customers are?
Dr. Christoph Mayer [00:02:09]:
Yes, roughly. Not by name, but we have a certain tilt towards newspaper publishers. We also work a lot for regional newspaper publishers, of which there are many in the German landscape. I'd say that's a large part of what we do. There's currently the issue of digital subscriptions, which keeps us very busy. Others are: We work for very large book publishers. And many of the customers we work for are actually large groups.
Christian Kallenberg [00:02:39]:
Okay, let's stay on the subject of subscriptions for a bit. The BDZV 2022 trend survey, which you initiated among others, was recently published, where the publishers surveyed said: "The biggest opportunity is growth through digital subscription models. But I've seen, so far that's only the case for a third of the respondents, that there's growth in digital subscription models. Is there quite a bit of optimism in that statement?
Dr. Christoph Mayer [00:03:09]:
Yes, you have to see where we come from a bit. The print business model was one that worked very successfully for decades. And if you think about it as a business model, it's a pretty ingenious model because you have extreme scaling with certain fixed costs. So we always talk about that when we talk about startups and stuff, how great scaling is and so on. You have to say the publisher business model is a pretty great one when it comes to scaling. Up until a couple of years ago, the thought was to actually offset the declines in print with new business models. That was also quite the trend. What a lot of publishers have done is build more things. Regional newspaper publishers have looked at: What regional expertise do we have? What do we have in terms of business contacts, in terms of trust among the population? What can we do with that? Magazines have looked at other things: What do we have in terms of cross-cutting issues? Can we make an app somewhere? Et cetera. And that was the big trend: diversification, building new business models. And that has recently changed significantly to the big trend: Maybe we can actually work much better with our journalistic expertise and bring what we do in print to digital. As you say, there is certainly a lot of optimism. But it's also driven to a certain extent by role models, especially from Scandinavia, which have simply shown that this switch is possible. When it comes to diversification and new business models, there have always been isolated examples, but there hasn't been a large group of role models. With digital, there are. So, yes, there is optimism there. Growth is high, but still at a comparatively low level. But I see this as an extremely exciting topic, and I would also find it extremely exciting from a journalistic perspective, if you really manage to continue to exist with your core, journalism, and don't have to start building any businesses around it, which are perhaps not so core.
Benjamin Kolb [00:05:12]:
"Persist" is perhaps the right keyword. You can see that the curves in print are still going down relatively steeply. And some people say that a certain part of the print business will continue to exist despite the falling curve, but at the moment it is still going very steeply downwards. At the same time, digital revenues are going up, but perhaps not to the same extent or certainly not to the same extent - and perhaps they don't have to, because print also involves more costs. So in order to keep the business model valid, you still have to make sure that it levels out again somewhere, which at least leaves you with a profit. According to these studies, it's always like this in five years, and that doesn't really change. Is the question: Is it therefore in five years because you simply can't foresee it yet, or what is your impression? So the gap is closing faster and faster and we are visibly approaching the end of the tunnel, or how do you see the situation?
Dr. Christoph Mayer [00:06:06]:
The fact that print circulation is declining is not somehow a new trend, but has been the case for 15 years - even longer. Yes, I don't know whether we should be so critical of that. Even a few years ago, there were statements like this: "Yes, in X years, if you continue to draw the line, it will be dead," et cetera. I don't really believe that. I think it's perhaps similar to the music industry. I used to have the Rolling Stones on a record, at some point the MiniDisc tried, didn't work out, came the CD, great success, MP3, streaming. In the end, it's a bit like the channel, the medium, which simply changes. That's why I don't think it's bad at all. It's simply a change in society, too, which is simply more accessible to other channels and other media. You're right, you always look a little bit into the future, five years, and see, they're always shifting et cetera. But I think we're currently in a situation where that seems quite realistic. Currently with the publishers, when we talk to them: "What is your target size for digital subscriptions? Where do you currently stand?", then it's usually somewhere in the range of: "We've reached about 5% to 15% of our target, but we have clear growth curves." It could well be that in five years, digital will really make the switch and will simply be the main medium in terms of production, technology, and so on.
Christian Kallenberg [00:07:41]:
Let's just stay with the trend survey. One of the top 3 trends mentioned there is personalization as a booster for paid content. How can even smaller publishers afford to invest in this trend? Isn't it insanely expensive?
Dr. Christoph Mayer [00:07:59]:
Vielleicht bevor ich auf die Frage eingehe noch mal: Warum finde ich das eins der spannendsten Themen aktuell? Und da komme ich auch auf die Frage: Was ist denn eigentlich das Medium und der Kanal? Und was verändert eigentlich der digitale Kanal mit dem Produkt? So ein Print-Produkt ist ja am Ende ein gebundletes Produkt. Das digitale Produkt ist nicht gebundlet, sondern das ist eher so ein Stream an Inhalten. Und das sind, finde ich, zwei sehr grundsätzliche Unterschiede, die man bedenken muss für den Erfolg im Digitalgeschäft. Beispiel: In Print hat jeder Artikel in diesem gebundleten Produkt grob eine ähnliche Chance, gesehen zu werden. Natürlich: Auf der Titelseite, auf den vorderen Seiten ist die Wahrscheinlichkeit höher. Aber einfach von der Nutzung des Produktes ist es ja so: Ich blättere das durch und vielleicht blättere ich es nicht zu Ende, aber mal ganz grob gesagt: Die Wahrscheinlichkeit jedes einzelnen Artikels überhaupt mal sichtbar zu sein, ist ähnlich. Im Digitalen ist das komplett anders. Ich kann dort einen Artikel auf die Top-Position vorne stellen und der wird immer gesehen und wird immer geklickt, oder ich stelle den unten auf die Seite oder auf irgendeine Unter-Übersichtsseite. Die Wahrscheinlichkeiten überhaupt nur gesehen zu werden, ändern sich dramatisch. Das ist ein wichtiger Unterschied. Ich erkläre noch mal gleich, was das mit Personalisierung zu tun hat. Der zweite, für mich wichtige Unterschied ist in dem Charakter des Produktes. Print-Produkt ist – na ja, für was bezahle ich? Ich bezahle nicht für einzelne Artikel gefühlt, sondern ich bezahle irgendwie für so ein gesamt gebundletes Produkt, eine Ausgabe. Die hat irgendwie auch so eine gewisse Wertigkeit. Das ist irgendwie so ein Packen Papier. Bei der Tageszeitung kriege ich das sogar noch frei Haus geliefert früh morgens. Bei Zeitschriften kriege ich das auch geliefert et cetera. Also das Produkt an sich hat so einen gebundleten Wert. Im Digitalen ändern sich diese zwei Dinge jetzt. Erst mal die Sichtbarkeit der Artikel ändert sich massiv und das Gefühl der Nutzer ist nicht: „Ich kriege da irgendwie für mein Geld sowas gebundletes. Das hat einen Wert in sich“, sondern die Qualität jedes einzelnen Artikels hat einen viel höheren Einfluss auf mein Gefühl, ob ich für mein Geld irgendeinen guten Gegenwert kriege. Jetzt ist die Frage: Was hat das mit Personalisierung zu tun? Der Effekt, dass zum Beispiel nicht jeder einzelne Artikel eine ähnliche Wahrscheinlichkeit hat, gesehen zu werden wie im Print, führt dazu, dass viele Nutzer auf die Seite gehen, sie sehen nicht sofort was für sie Spannendes und gehen wieder. Und nur sehr wenige Nutzer scrollen überhaupt relevant weit nach unten. Wir hatten das jüngst in einem Projekt im Detail mal gemessen. So ganz grob, wenn ich hier so an meinem Laptop bin, öffne die Seite – ich gehe zwei Bildschirmseiten nach unten. Wie viel Prozent der Nutzer kommt überhaupt so weit? Ungefähr ein Drittel. Das heißt, zwei Drittel habe ich dann schon verloren, weil sie nicht direkt auf dem obersten Teil was Spannendes für sie gefunden haben. So, jetzt kann ich sagen: „Na ja, das steuere ich ja selber als Redaktion. Ich packe halt oben immer was Spannendes hin“, aber spannend ist halt für jeden Nutzer auch irgendwo was anderes. Deshalb ist das, finde ich zum Beispiel, ein Punkt, wo Personalisierung extrem wichtig ist, dass ich sagen kann: „In den oberen Viewport, wo ein Nutzer kommt, stelle ich sicher, dass für ihn persönlich was Spannendes da ist.“ Das hat, glaube ich, einen riesen Effekt auf Nutzungsverhalten, damit ich dann eben nicht draufgehe, sage: „Da ist ja gar nichts Spannendes“, und ich gehe wieder. Das hat eben, finde ich, viel damit zu tun, wie das digitale Produkt und das Print-Produkt einfach einen ganz anderen Charakter haben. Jetzt zu deiner Frage bezüglich Kosten und teuer: Auch bei Personalisierung gibt es ja unterschiedliche Ansätze. Ich kann sagen: „Ich habe eine vollpersonalisierte Seite. Da ist alles hochindividuell.“ Das ist ja das, was wir von Netflix, YouTube und Co. gewohnt sind. Ich glaube, das braucht es gar nicht. Das braucht es, glaube ich, erst mal deshalb nicht, weil so viele Inhalte, dass so etwas notwendig ist, haben die Verlage ja meistens nicht. YouTube und Netflix und Co. sind ja gezwungen, zu vollpersonalisieren, weil das ist ja wie so ein Eisberg – oben sichtbare Fläche auf dem Portal sehr gering im Vergleich zu der riesen Anzahl an Inhalten, die es gibt. Und dann bin ich gezwungen, Vollpersonalisierung zu machen. Die Publisher sind dazu eigentlich nicht gezwungen, sondern sie können am Ende einen Mix davon machen und sagen: „Ich habe gewisse Positionen, auf denen ich eben personalisierte Empfehlungen für den einzelnen Nutzer ausspiele.“ Oder Personalisierung heißt ja auch schon, dass ich sage: „Eigentlich auf der Startseite sieht jeder die gleichen Artikel, aber jeder in einer anderen Reihenfolge.“ Also was für dich ein spannender Artikel ist, ist weiter oben. Ich finde den vielleicht nicht so spannend – für mich erscheint der vielleicht weiter unten. Und ich glaube, dadurch dass man ein Stück weit Abkehr hat von so dem Gedanken Vollpersonalisierung, hat das einen massiven Einkurs auf die Kosten. Aktuell ist eher die Herausforderung noch: Es gibt eigentlich wenig Branchenstandards, die ich einfach Plug and Play nutzen kann. Vieles von dem, was es gibt, kommt so ganz stark aus dem E-Commerce getrieben. Meiner Meinung nach ist Personalisierung für News-Inhalte was anderes, wie wenn ich dir Turnschuhe empfehle. Beispielsweise alleine schon weil News-Inhalte, mindestens wenn sie im Tageszeitungsbereich sind, sehr schnell altern. Ich muss manche Artikel dir übermorgen gar nicht mehr zeigen. Da sind die schon überholt und veraltet. Der Turnschuh hat ein bisschen eine längere Lebenszeit. Das macht, finde ich, die Komplexität im News-Bereich höher und ist bestimmt ein Problem, weil es einfach nicht Out-of-the-Box-Systeme gibt, die ich anschließen kann, sondern eigentlich ist das heute noch für jeden so der eigene Weg, den er beschreiten muss, sich da eine Lösung zu bauen. Deshalb hast du ein Stück recht. Aktuell sind die Kosten noch dafür wahrscheinlich für einige hoch.
Benjamin Kolb [00:13:44]:
Yes, of course we can confirm that the complexity of a recommendation engine in the area of news or articles is generally greater than in the e-commerce area, but it's not an insurmountable problem. I think that with our own recommendation engine, which is available at Purple, for example, where it is relatively easy to import content from any system and simply play out the recommendations again, i.e., which can also be integrated relatively easily into websites, we can achieve a fairly exact match by having exactly these switches available. So what role does the topicality of my content actually play? How strongly does it have to be thematically related, what is actually recommended and so on? These are basically all sliders that you have to actually set your recommendation for your product, because what is also clear: Not every brand ticks the same. So, of course, a global news publisher is different from a regional news publisher, is different from a magazine brand, and so on. That means you basically need all these adjusting screws in order to see the type of content reflected in the recommendations. I completely agree with you on that. But I wanted to mention one other thing, which I find totally exciting, is your comparison or the differences between the print and digital product, because one exciting question that I always ask myself is: Why is the trend of this e-paper going up and up? Why do users actually read a print product in digital, which is in principle totally impractical? What I could imagine - and I don't know if you can confirm this - is that as an editorial team, and above all of course as the editor-in-chief, but also as the editorial team as a whole, you can say at some point: "This is what we're printing," and that naturally requires a certain amount of pressure to achieve a certain quality. And this is then actually printed, which means that it is edited in a completely different way than an online article, where even with the very large and actually high-quality news publishers in Germany you also find spelling errors and everything that are simply, let's say, inferior features. You don't normally find that in a newspaper like this, because it's printed, and of course it's a huge investment to print something like that. Once it's out, it's hard to correct things, so it's a different quality, and it has the character of a bundle, which is what you meant. That is, as a user, I also have the feeling that at some point I'll be through with this news situation and put it down and have the feeling: "So, now I'm informed for the day and don't have to deal with it again." I think the most frustrating thing about digital news apps like that is actually: you open it, then you open it again, then you open it again, and somehow you don't even know, "What of this whole content stream have I seen eight times now? And what's actually new?" So I never feel like I'm done in it or somehow I've read a meaningful package that somebody, as it is in the newspaper, has manually put together for me also with an intention of the brand behind it. What is your perception of that?
Dr. Christoph Mayer [00:16:49]:
So e-paper is really extremely exciting. We've come from a time when people said, "Well, we have the printed product and a PDF just falls out of the production process." And then at some point they said, "Maybe people would be interested in just looking at this PDF." And for years, it was really only for a strange niche clientele that actually wants the newspaper, but for some reason they also like the PDF.
Benjamin Kolb [00:17:17]:
Yes, or want to read on vacation as well.
Dr. Christoph Mayer [00:17:19]:
Exactly. And everybody actually assumed that: At some point, this will take care of itself. It doesn't at all. E-paper is a huge trend. And that, I think, has exactly to do with what you're saying: I'll be finished at some point. I think Corona had had its share of that, too. People are so tired of it to some extent - news fatigue. And as you say, I go to a site - that ends up being an endless stream of information. News tends to be a bit negative as well. It's not the latest great information that the world is doing much better than it was X years ago, and another success story here and a great story there, but it's also primarily more negative content. I think that's a big problem, and maybe we can talk about it later. But that leads to this: In the end, if I look at it dryly, I have an endless stream of negative information. And the e-paper offers a very good opportunity to simply say: "I've now leafed through this thing. That's it for today. I'm done."
Christian Kallenberg [00:18:22]:
Yes, I can confirm that again from my side. In the last episode of this podcast, I spoke with the Chairman of the Supervisory Board of Axel Springer, who also confirmed once again that his favorite form of media at the moment is the e-paper, for the very reason that it is bundled, it has a beginning, and it has an end. Nevertheless, let's go back to the BDZV trend survey. The e-paper is one of the various output channels that can be seen: Okay, print is now perhaps only read by the very old, e-paper is read by the middle-aged, online is read by another generation younger, and podcasts are read by the very young. Is that also your perception, or do you think it's already become outdated again, that the output channels can be arranged according to age groups in this way?
Dr. Christoph Mayer [00:19:12]:
I think, very roughly, that's true. I don't think the print age structure will change much. I don't think we'll see a trend where print readers will become significantly younger again. With e-paper, I have the feeling - for the reasons we just discussed - that younger age groups are also tending to say, "Hey, that's exciting somehow." E-paper also has a lot of opportunities to prepare it on a tablet in a different way than just a scrollable PDF. So I think with paid, web paid and e-paper, they're shifting a little bit towards each other. I think e-paper will tend to be a bit younger. Paid is getting a bit older. I think those are the two movements there. Print is becoming the target group that it has. And you also have to say that 80%, 90% of publishers' revenues still come from print. So we always talk a little bit about, "Yeah, this thing is kind of through," but that's not the case in any way. But I think it's going to stay that way in its age group. I think those are the movements.
Christian Kallenberg [00:20:12]:
Let's just stay with the target groups. There is not only the option of mapping them according to demographic factors, but also as defined in the trend survey - according to need groups. What's that all about?
Dr. Christoph Mayer [00:20:24]:
We've all heard the model, "Let's think about personas." That's the classic, which also came into the newsrooms at some point. "Let's think about who our readers actually are," and usually there are three or four personas. There's Max, who's 30 and likes snowboarding and lives in the city, and then there's Franz, who's 60 and almost retired, and so on. What you actually notice when you look at the target groups: That's a good first step to even get into a mindset of saying, "Hey, we're writing for someone. We're not writing for ourselves." Or this idea: "We have such a chronicler's duty," that also exists in part or used to exist increasingly in newsrooms, that people say, "Our duty is simply to write things down so that they somehow end up in such a chronicle in such an archive." From my point of view, a very absurd idea. And personas are such a first step to even say, "Oh yes, there's someone we're writing for." But you realize relatively quickly that these personas don't exist. Max doesn't exist, Franz doesn't exist, but somehow they've come up with something that's usually based a bit on clichés. And then there are other ways of thinking, of saying, "Well, what is there?" There are, I'll say, interest groups, audiences, there are people who watch what they eat and want to live healthy. That's maybe a target group, that kind of exists. It doesn't have so much to do with age or anything else, it's more like a bit of a cross-section of the population. And more and more newsrooms are moving in a direction where they are saying, "Yes, let's think about it, if the personas don't really exist: What are some cross-sectional audiences like that that we can use when we're writing content?" And then there's a way - that's actually the right way from our point of view, but it's also a bit more complex to implement for the newsroom. It's called, "Let's go in the direction of needs." Not: "What is the cross-section of interests in the population?" but: "What are actually needs of people who have them?" User Needs - there's such a quite famous model from the BBC, for example. Six User Needs. That is then for example: What are people's needs? Security is a need of people. I want my personal security. I'm concerned about that, but I'm also concerned mentally about security: what's going on in the world anyway? I want to be up to date, I want to learn things. That's another step, preparing content, writing, structuring a newsroom that is geared to people's needs.
Benjamin Kolb [00:23:05]:
In addition to the needs-based approach, which is another step further into abstraction, there is also the behavior-based approach. So what kind of readers are they? Are they those who look at the page for ten seconds in the morning or those who take a lot of time in the evening? That's certainly something that's taken into account in the newsroom, too, and that's how the work is designed to a certain extent. How much of a trend do you see, that people don't necessarily try to imagine it, but rather have a machine determine what the target group is? So the question is a bit: Why don't we try to let machines figure that out? For example, by clustering certain interests or user behaviors, by clustering interests, and by trying to connect this to learn from this, in principle, what the user groups of my brand actually are, and perhaps derive from this what their needs are.
Dr. Christoph Mayer [00:24:04]:
So we do a lot of analyses. Maybe we'll come back to the DRIVE project later, which we're doing, where we're doing a lot of analysis about online usage behavior, about content, and so on. One point where analyses always end is the problem: I only do a certain kind of thing, so in the end I can only measure today what I already do as a publisher. If I never have certain types of content on the site, then I'll never be able to measure whether there are people who would actually be interested in such content. Or if I never actually put new, fresh content on the site early in the morning, I'll never know if they would like that if there's content there. So from my point of view, that's a limiting factor: I can only ever measure what I'm already doing to a sufficient extent. The exciting thing is that when you work with several publishers - each one of them is perhaps also doing a certain kind of process or content or something by chance. So that's where the opportunities come from. When I sort of take that a little bit bigger and have the ability to think outside the box data-wise, then all of a sudden I see things that somebody else might also just be doing by accident, and I can measure that. I think the problems and challenges that publishers face are still a notch simpler most of the time. So I don't think we need the huge complex AI models and stuff, but if I just look at: What is the content that works well? And those that don't work well - I simply put them in two buckets and read a bit about the articles, then it usually becomes clear relatively quickly where the problem actually lies, namely content where, for example, I simply list facts - if it was perhaps in a regional daily newspaper: local council meeting and it was about the construction of a new school. And now I can write that in a variety of ways. And what we see: If I just dryly write the facts there: "And a great school is being built now and it costs 1 million and another 200,000 for the sports field and we're 5% over budget," then people aren't really interested in that in digital. But if I write about the background: "And how did it actually come about? And what do the residents say about the new school? And what is the situation like? Do we have too few schools in the region at all? What do the parents say?", et cetera, then that's a different way of writing about it, and we see that such content works much better. I think you're absolutely right that the right approach is to analyze that with algorithms at the end, to understand. To do that, I have to publish a bit of diversity like that as well, so that I have enough ways to measure in the first place. I think with a lot of newsrooms, the problem is still at a level where maybe that's not even necessary yet.
Benjamin Kolb [00:26:51]:
Now you've already mentioned it yourself - DRIVE, the project. Totally exciting. Can you explain to us briefly and succinctly what that is?
Dr. Christoph Mayer [00:27:00]:
I think you can explain the project well if you look at how it came about. Just before Corona, we had a lot of conversations with managing directors, editors-in-chief, digital managers in publishing houses: "What are you actually planning? What are you doing to strengthen your digital business?" And at the time, everyone looked to Scandinavia to publishers like Schibsted, Amedia and the like, and everyone said, "Well, we'll see what they're doing over there. They are working extremely hard with data to understand user behavior, to understand: What kind of content do users actually want? How are they reading the content? When do they come to the site?" Et cetera et cetera. Many have said, "We want to do exactly the same thing. We want to understand with data and align our product and newsroom based on that." When asked, "Where do you currently stand?" most have said, "Well, we're going to see if we can get a data scientist hired like that." Some have also said, "We've already tried that and it's not really working out. We can't find the people. And when we do have someone, he or she doesn't have an easy time as a lone wolf in our organization." And that's where the idea came from, together with the dpa: If many are faced with the same issue and our focus is on regional publishers who are actually only in competition in peripheral areas - all have similar issues, all have the same challenges on a technical, personnel level, then let's do it together. And that's how the DRIVE project came about. It's now 20 regional newspaper publishers in Germany and Austria. And what we do: We collect very fine-grained data directly from the websites. We store every user interaction in the data warehouse that we build and manage at Schickler. Every published article flows into it, and we answer all the questions that the newsrooms have together in a wide variety of working groups in a process that is very transparent internally, where we conduct experiments, A/B tests, for example, in which several of the publishers then participate. We control this, we bring in the analyses, we bring in our data team, which analyzes user behavior on the pages, which analyzes articles, and so on. I always call it large scale experimenting and shared learning, where we think: Together, we're making faster progress than anyone could on their own. Why? Well, not everyone has to participate in every analysis and every experiment, but we have some experiments and working groups where maybe only three publishers say, "This is an exciting topic for me. I want to participate in that." But everything that we generate in terms of fundamental insights is accessible to everyone again in the group. And this creates speed, because I can benefit from many more experiments and analyses than if I were to do it all myself. I think we have a lot of expertise that we bring in there, not only on the data side, but also to structure these questions, to make analyses, to moderate these groups. That is the DRIVE project. And in addition to analyses, experiments and findings for the newsrooms, we are now increasingly moving in the direction of analyzing the data that we have at the article level, at the user level, where we predict scores, purchase probabilities, churn probabilities, other things - we also return this to the respective publisher for its articles, its users, via APIs, so that it can use it in other systems, for example in the CAM system. This goes so far that we also develop algorithms for personalization, as we briefly mentioned earlier, not just from a purely technical perspective, but in joint working groups with publishers, with experts from the various newsrooms, also very much with the question: "What do you actually want? After all, it's your product. What is your idea of how personalization should work?" And we at Schickler then develop that in the form of algorithms and the like, and make it available to the publishers via interfaces. Yes, and so this is such a large, joint project with now 20 publishers - in total, over 200 participants who come from the publishers. I would say 60 to 70, who are working very intensively in the various working groups, and where I think that is really the idea that we originally had. They all have very similar issues and we just move forward much faster together. That has absolutely come true.
Christian Kallenberg [00:31:33]:
And DRIVE stands for Digital Reader Revenue Initiative. Is that right?
Dr. Christoph Mayer [00:31:38]:
Exactly. But I think we were more looking for a catchy name and then thought about it afterwards: What could it actually be called?
Christian Kallenberg [00:31:45]:
Yes, sounds great. One of the big findings of DRIVE is the definition of the new KPI, namely media time. Could you explain that briefly?
Dr. Christoph Mayer [00:31:55]:
Also alles, was wir bei DRIVE an Analysen, Metriken und Co. machen, entwickeln wir dort gemeinsam mit den Verlagen. Und eine der ersten Arbeitsgruppen – ich glaube, es war die erste Arbeitsgruppe – war: Was wollen wir eigentlich verbessern? Jetzt sagt jeder: „Na ja, wir wollen mehr Digital-Abos.“ Das ist aber keine Metrik, die man wirklich nutzen kann, um zum Beispiel einen Newsroom zu steuern oder um zu messen, ob jetzt gewisse Inhalte besser oder schlechter funktionieren, sondern die Abos sind am Ende ein Ergebnis. Das ist nicht die Metrik, auf der ich sinnvoll steuern und Entscheidungen treffen kann. Deshalb hatten wir dann eben die Frage: Was ist denn eine sinnvolle Metrik? Und wir kommen ja alle aus einer Welt von Visits, Unique Usern, Page Impressions et cetera, wo wir aber schnell das Gefühl hatten: Für das, was wir hier tun, mit einem journalistischen Anspruch, passt das nicht so richtig, weil ein Seitenaufruf kann extrem unterschiedlich sein. Das kann sein: Jemand verklickt sich kurz, kommt von Google und ist danach wieder weg. Oder es kann jemand sein, der einen Artikel aufruft und den fünf Minuten lang intensiv nutzt. Beides Mal den Seitenaufruf als 1 zu zählen, ist irgendwie ein komisches Gefühl. Das passt nicht so richtig. Wir haben dann viel darüber diskutiert, was sinnvolle Metriken sein könnten, und sind am Ende relativ simpel bei der Zeit gelangt. Also wir messen für jeden Nutzer: Wie viel Zeit gibt er uns? Also jetzt technisch schauen wir uns an die letzten sieben Tage: Wie viele Minuten, Sekunden war er denn in Summe bei uns auf dem Portal? Das heißt, jedes Mal, wenn eine Session startet, dann läuft die Uhr, wenn er geht, hält sie an, und wenn er wieder neu kommt, dann läuft sie weiter. Also eine kumulierte Zeiteinheit auf Nutzerebene. Das war damals erst mal so eine fixe Idee. Wir haben das dann in das Tracking integriert, haben erste Analysen gemacht, waren sehr erstaunt, dass wenn man dann daraus Nutzersegmente baut, wir dann gesagt haben: „Na ja, letzte sieben Tagen – also so ein, zwei Minuten muss der Nutzer schon irgendwie da gewesen sein, ansonsten ist das schwierig.“ Und dann haben wir zum Beispiel mal geschaut: Wie viele Nutzer sind denn eigentlich bezogen auf die letzten sieben Tage länger als zwei Minuten auf der Seite? Das waren dann 5% der Nutzer, was uns dann gezeigt hat: „Hey, diese hohen Zahlen von Nutzern, Unique Usern und Co., wo ja alle immer ganz stolz berichten, wie viele Gesamtnutzungen, wie viele Visits und so weiter – die Zahlen sind völlig irrelevant. Die Zahlen sind so aufgebläht mit für uns – aus Sicht von Paid Content – irrelevanten Nutzermengen“, dass das uns noch mal bestärkt hat, dass Media Time sinnvoll ist. Und was ich besonders spannend finde, ist: In der Zeit stecken ja verschiedenste andere Metriken drin. Also erst mal steckt dort drin: Wie häufig kommt jemand? Und wenn er kommt, wie lange bleibt er? Wie lange er bleibt, da steckt natürlich drin: Wie viele Artikel liest er eigentlich? Und da steckt auch irgendwie drin: Wie weit scrollt er dort runter? Wie intensiv liest er die? Also es ist am Ende für uns so eine Obermetrik, die ganz viele Aktivitäten darunter beinhaltet. Wir kamen dann irgendwann zu dem Punkt: „Na ja, wir können das ja nicht nur für jeden einzelnen Nutzer messen, sondern lasst und doch mal auf Artikelebene messen. Also lasst uns einfach mal die Gesamtnutzungszeit auf diesen Artikeln – also die Anzahl der Nutzer mal die durchschnittliche Zeit ist das dann am Ende – lasst uns das doch mal messen.“ Und was heißt das auf Artikelebene? Das ist dann auch die Metrik, die wir uns auf Artikelebene jetzt anschauen. Das ist dann so ein bisschen: Na ja, dieser Artikel hatte 30 Stunden Nutzungszeit. Oder wir schauen uns das auf Gesamtportalebene an. Das gesamte Portal hatte in den letzten sieben Tagen 500 Stunden. Und geht das hoch oder geht das runter? Et cetera. Das ist aus unserer Sicht eine sehr gute Metrik, weil sie ist keine so Clickbait-Metrik, wo ich einfach nur viele Seitenaufrufe möchte, sondern es hat was mit der Qualität der Nutzung zu tun. Und wir hatten in ersten Analysen gesehen, dass die Media Time Nutzungsintensität auf Nutzerebene ganz stark mit Conversion- und Churn-Wahrscheinlichkeiten des Abos korreliert. Das heißt, wenn ich es schaffe, dass der Nutzer das Produkt stärker nutzt, dann steigt automatisch die Wahrscheinlichkeit, dass er in das Abo reingeht. Und deshalb: Wir sagen, für den Newsroom ist Media Time die Kernmetrik. Schreibt Inhalte so, dass die Nutzer sie am Ende gut finden, dass viel Media Time entsteht. Dann ist es die Aufgabe, die Paywall so zu steuern, den Bezahlprozess und das Gesamtprodukt, Pricing und Co. so attraktiv zu machen, dass ich diese Media Time dann umgewandelt kriege in ein Abo. Das heißt dann auch für uns, dass wir dem Newsroom nicht irgendwie ein Dashboard hinhängen und sagen: „Ziel ist heute, 50 Abos zu machen. Ihr braucht aber noch zehn. Gebt Gas.“ Dann schauen die uns mit großen Augen an und sagen: „Was sollen wir denn tun genau? Was soll ich denn als Redakteur genau tun, damit ich jetzt von den 30 Abos heute noch auf die 50 Abos komme?“ Und so ist das eine andere Diskussion und eine andere Metrik, weil ich kann sagen: „Was sind denn eigentlich Best Practices, um Media Time zu generieren bei den Nutzern?“ Und alles an Experimenten, Inhalte anders aufzubereiten, anders zu schreiben, Themen et cetera, fokussiert dann bei uns auf Media Time, wo wir dem Newsroom halt wirklich sagen können: „Diese Art der Aufbereitung von Inhalten sorgt dafür, dass die Nutzer sie gut finden und dass sie sie intensiv lesen.“
Benjamin Kolb [00:37:06]:
Are there any, let's say, generic findings at this point as to what increases media time in principle? What influencing factors would there be? Telling the editorial team, "Now increase the media time," is not such a dedicated instruction that they can simply implement. You can't always just say, "You have to produce more quality now," or "The stories have to be better. The question is: Are there a few other factors that you can take as a basis that influence this?
Dr. Christoph Mayer [00:37:32]:
So, for example, we did an experiment, I think with three or four publishers, where the question was: How do I actually write good teasers? That actually came about because one publisher said, "Hey, we're discussing ourselves to death there in the newsroom. Everybody kind of has a different opinion on how to write teasers." Some say as lurid as possible, mysterious, so the user will click into the article. The others say it should be as good a short summary of the article as possible. And the third say, "I don't care at all. I'll take the first two sentences from the article and that's it." For many publishers, this was an issue where they said, "Somehow this is annoying. We're arguing like fools there. Can't we analyze together at DRIVE how to write good teasers? Because we think that's the showcase for the article, and we want people to read the articles, because if nobody clicks into the articles - nobody's paid money for the overview page yet." And we had big experiments, A/B tests, just to see: What actually causes people to click into the article? We saw huge differences in the way you write teasers, sometimes up to a factor of 2 higher click rates. And that, for example, is a finding that we then prepare together with the editors-in-chief: These are somehow the seven rules for writing good teasers. Why is that relevant for Media Time? Well, if the users don't go into the articles, then they don't read any articles, then there isn't enough usage time. And we know from analytics, then no subscription is created. Other very simple things: How big is actually the effect of subheadings? So I have a long article - some editors say, "I always structure it and put in subheadings," others say, "That's too cumbersome for me. If the user likes the article, he'll read it." We had a smaller working group where we analyzed in an A/B test: How important are subheadings? Relatively simple. One part of the users saw the normal page with subheadings, for the others we hid it. And we were able to measure: How does the intensity change for users in terms of media time? And were able to say, "Look, this is how high the effect is." After how many paragraphs does a subhead make sense? Et cetera. What I mean to say is: in part, it's also craft things that simply pay into the product. Other findings are then on emotion structures of articles, on a topic level. Which topics manage to pay off on media time and how strongly? On a length level: if I'm an editor - should I write two short articles or one long article? And so it's different frameworks at the end and input for the newsroom to improve its work.
Benjamin Kolb [00:40:09]:
By the way, that's also an exciting difference between the digital world and the intermediate step of an e-paper: You don't have any clickbait teasers standing in between, but you can see at a glance, so to speak, what the overall situation is, which is perhaps also an exciting element in the whole story.
Dr. Christoph Mayer [00:40:25]:
This is extremely interesting. If you've taken a look at the new features on Netflix and YouTube in the last few weeks, or maybe months, you'll see mouseover autoplay. And I think this has exactly this background, to say: How do I actually get, if the user has even a little bit of interest, which means he goes with the mouse over the little picture of the video - to pull him into the article. And you're right, with print, with e-paper, I don't have that problem at all. I can see the content immediately, so to speak. Another interesting question: What kind of evolutionary steps should news publishers actually take on their home page? And is something like this perhaps also an exciting topic? Once with the mouse over it and the intro text becomes a tad longer.
Benjamin Kolb [00:41:09]:
Actually avoiding the disappointment of a misguided click. It's like when you have a clickbait teaser and then you read the article where the story is pretty thin at the end - it's just not fun. You then expect quality behind such a click that you do, which then comes.
Dr. Christoph Mayer [00:41:23]:
Yes, perhaps one more thought on this in the direction of personalization. When I think about "What do I optimize for in personalization?", the basic logic is to say: "Well, I optimize for clicks. I just want him to click into the thing." But as you say - one disappointed click - I quickly conclude, "Probably everything else on the page is not for me either," and leave. That's why if you look at how YouTube, for example, does personalization - very, very simplified - I mean, it's also only part really public, but what's public: in the end, it's three metrics that they optimize on. The likelihood of someone clicking on it, the expected duration of how long someone watches the video, and then: What's the likelihood that I'll continue to stay on the portal after watching the video? Or is it the disappointed click and I leave? And from these three metrics, for each combination of user and video, I'll say, there's an expected additional usage time not just of the one video, but of the entire session on it, so to speak. And I think that's very exciting thinking, just not just saying, "Well, I'm optimizing for the click," but also saying, "Well, how likely is it that if the user clicks on this article, they're going to read more or go afterwards?" This thought process aligns quite well with our media time metric. Open parenthesis: Something like YouTube, Netflix et cetera, of course, work at their core with something like watchtime, which is actually also our media time, and so fits a bit into this overall world of thought that we also follow.
Benjamin Kolb [00:42:53]:
What has actually happened in this DRIVE project, for example, in the area of data science? How much AI technology are you using there? Can you go into that a little bit?
Dr. Christoph Mayer [00:43:01]:
So what we do at DRIVE is that we build a large data warehouse in the cloud and the whole tracking pipeline and so on, in order to be able to collect fine-granular events from all sides in a structured, uniform form. The other things that will flow in are pipelines for articles and so on. So that is first of all the goal in the connection of a publisher, to collect all relevant usage events with high quality and high information content. This means, for example, that we get events not only when someone calls up an article, scrolls down, etc., but also when they run up against the paywall, for example, or when they click on the offer on the paywall and say, "It's interesting," so that we can also measure the entire payment funnel, etc. A lot of that is engineering issues, building a system like that in the first place that can collect data accordingly with quality and scalable. And then we get into the whole topic of how do we process, analyze data? A first AI model is, for example, the automatic tagging of the articles in different dimensions. This is also, for example, a structure that first emerged in the working group: What is a topic structure in the first place that offers publishers added value? There we developed a topic structure, an emotion structure, such a genre structure et cetera and first said, "Hey, what actually brings you added value in the newsroom if we build analyses on which levels?" These are then, for example, models that we have trained ourselves in the end, because there is nothing pre-trained, so to speak, on precisely this structure. And when a publisher publishes an article, it automatically flows into the data warehouse and is automatically indexed in these various dimensions. That's an AI model, for example, that's running there. Others are in the area of propensities, predicting user buying behavior. What is the likelihood that this user, if we show them a great subscription offer now, will buy that offer? Or personalization algorithms, where we work a lot with NLP algorithms to make articles so machine-readable that we can use algorithms to make predictions for users: Which articles actually match the topic interest of these users? These are different AI models. Many exciting analyses do not necessarily have anything to do directly with machine learning and the like in the narrower sense. One of the most exciting topics we are currently working on, for example, is what we call "traffic corrector. In other words, the possibility of adjusting article performance for positioning effects. If an article is at the top of the home page, it will always get traffic, no matter how good or bad it is. If I bury a great article somewhere on the page and hardly anyone can see it, then it gets little traffic. And now this is not so trivial, because the articles wander over the page. It starts at the top, then it's there, then there, then there. So the positioning changes and it has further effects on which day of the week and which time of day I am where. If I'm at the top of the home page, I always get traffic, except at three in the morning. I get relatively little traffic there. So there are also time-of-day effects. And we've made models and analyses to measure this fingerprint, so to speak, of every portal that's on DRIVE so that we can calculate these positioning effects out of the article performance. This is an extremely analytically and technically demanding topic - not necessarily machine learning - but from my point of view extremely valuable, because it gives us the opportunity for the first time to be able to measure article performance free of positioning effects. And positioning effects are huge. That is, hypothesis: if a publisher looks at a ranking today and says, "This was the best article today," the probability of that being wrong is very, very high.
Benjamin Kolb [00:46:54]:
From your point of view, what is the most exciting finding of the DRIVE project so far? As far as I understand it now: The DRIVE project is continuing. More and more publishers are now on board, which is of course great from a data perspective, because the more data, the more insights you'll probably gain. But what has been the most exciting insight you've come up with so far, one that has perhaps really surprised you?
Dr. Christoph Mayer [00:47:14]:
It's difficult to focus on one topic. For me, at the very beginning - it's already been a year and a half - this realization: "Which users are actually relevant for us?" was a very exciting one, where I say: "In the end, that's somehow 5% of the users. And let's move away from these big numbers. Not millions of unique users, millions of visits et cetera." Those end up being numbers that actually just distract us from what's really important. And what are actually metrics to really narrow down: Which users are really exciting for us now? How many are they? Which ones are they? How do they behave? I found that to be a very exciting topic in the overall context, because what we do, for example, is we simply filter a lot of the analyses that we do to these users that are relevant from our point of view, that is, somehow an article or a topic where I filter out all the usage that is actually just byproduct, and focus on the users that are really exciting. And then, I find, some numbers turn completely around, where before you say, "Wow, that totally interests the users," you now have to say, "Yes, that didn't interest them at all. There was just a big swing from Google News coming over." I think that's an extremely exciting topic. This, what I just said, traffic position shakeout I find extremely exciting. Another topic that I personally find very exciting is: we built attribution models for conversion, because in most newsrooms there are dashboards that say: "Look, this article made ten subscriptions today." And we've built attribution models to say, "What is actually the contribution of each individual article on the way to a subscription?" And there you have to say: that has little to do with the last article. I always compare it to a soccer game, where at the end of the game you say, "Everyone who didn't score a goal today won't be there next game day, they'll go to the bench." Then you have to look at what kind of team you have standing there on the next matchday, and you'll realize, "Maybe wasn't such a good decision after all." And that's partly how it is in newsrooms, when people say, "Only the articles that got a subscription are really good." And that's not true, but in attribution modeling we see: Lots of articles. And then - because, again, we do the analytics on groups of articles that have a certain theme or have a certain emotion or genre - we see that those are actually the game changers. Like in soccer, I have those who prepare the goal and those who make it. And those are two different types of players in the end. And I find this analysis and the possibility of making such analyses one of the most exciting topics, so that we can get away from always just looking at the goal scorers and claiming that I actually only need goal scorers and strikers on the pitch, which is simply not correct, but rather I need a meaningful interplay of an entire team in the end.
Christian Kallenberg [00:49:59]:
Christoph, we've now talked a lot about Media Time and how you can perhaps get users to sign up for a subscription through personalization at the end of the day. Now let's turn the focus away from the consultant, Christoph Mayer, to the end customer: Who was able to persuade you to take out a subscription to their service?
Dr. Christoph Mayer [00:50:19]:
I pay for the digital subscription to the regional daily newspaper in the city where I live, in Hamburg, I pay for Netflix, and I think that's where it honestly ends. I try out a lot, but it's more of a professional interest, so I often take out a subscription somewhere, either to a national magazine or to a regional daily newspaper somewhere completely different in Germany, just to see: What is the user experience like there? What is the payment process like? Is there any kind of onboarding campaign that goes on? Et cetera.
Christian Kallenberg [00:50:56]:
Crazy: Do you still read anything in print? So do you pick up anything besides the tablet or any other screen?
Dr. Christoph Mayer [00:51:02]:
Honestly, only when I'm at the airport or on the train and I benefit from other sales there, so free display.
Christian Kallenberg [00:51:12]:
And maybe no reception - at least on the train.
Dr. Christoph Mayer [00:51:14]:
Exactly, yes.
Christian Kallenberg [00:51:15]:
Christoph, thank you very much for your time. Very interesting. And toi, toi, toi for the further success of DRIVE. I'm curious to see what else will come out of it.
Dr. Christoph Mayer [00:51:23]:
Thank you very much.
Benjamin Kolb [00:51:24]:
Thank you very much, Christoph.
Dr. Christoph Mayer [00:51:25]:
Thank you.
Christian Kallenberg [00:51:27]:
And if you, dear listeners, enjoyed this episode, I would be happy if you subscribe to us on the podcast platform of your choice.